An Introduction to Data Lobbying
In my first newsletter post, I shared the unifying theory that organizations and individuals should care about data1 because it can be used to improve decisions. This can be easily illustrated by imagining a world without any data at our fingertips. Movie nights would be a special sort of hell. We wouldn’t be able to compare pizza restaurants or the movie options—and since prices are a type of data—we have no idea what we’d pay for either!
Data can improve decisions. I can pick one pizza place over another because it’s rated more highly on a website I trust, or even better, the website tailors a recommendation to me because it has some knowledge of my preferences through prior interactions on the site (e.g. my willingness to try the cheapest or most expensive options). I could rely on data incorrectly, however. It’s possible that after reviewing the data available I don’t end up picking the pizza place or movie I’d have liked, or wanted most. By in large, most people have an intuitive understanding that adding hard data to their decision-making process improves the results.
As a result, I think many, from someone who just wants a good pizza2, to the tech executive deciding what new feature to build, end up misusing data. They inadvertently make the logical leap from the fact that data can improve decisions to assuming that it always does, or more specifically, using any data that appears relevant to a decision will improve the resulting decision. These people are prone to data lobbying.
Data Lobbying: When an entity (e.g. individual or organization) presents data to another with the purpose of influencing the data presentee to make a decision that benefits the data presenter.
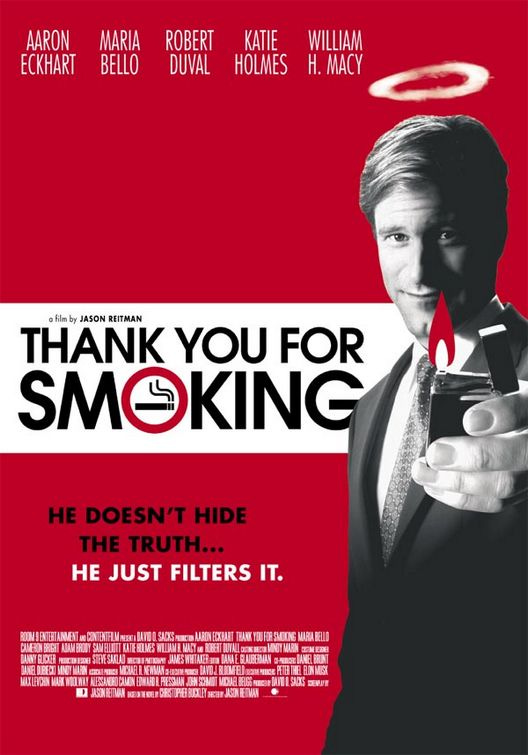
We need look no farther than the world of advertising to see data lobbying at work. Lottery ads present data in a way that benefit the lotteries: they often feature prior big winners and play up the amounts won (e.g. “George won $8M!”), letting the viewer imagine themselves in that position. A subset of those viewers will enter the lottery because they saw the ad. It’s important to note that what the advertisers are doing isn’t fraudulent. The person and winning amount they advertise are real, and a future winner winning the same amount may very well be possible.
Alternatively, the lottery operators could have advertised another datapoint, “99% of people who enter our lottery lose money.” It’s also a true claim, but far less likely to persuade folks to buy lottery tickets. We could imagine that some folks buy lottery tickets regardless of what ads they see, or even if they see no ads at all. On the flip side, some people will never buy a lottery ticket. Of the remaining folks, ones who may or may not buy a lottery ticket depending on what they see, I’m most interested in this group: those who buy a lottery ticket if they see the ad about George winning $8M, but not when they see the ad about having a 99% chance of losing money. In this simple example, these are the people who are affected by3 data lobbying. One of the two ads gets them to engage in the desirable behavior (from the standpoint of the lottery operator), and the other prevents it.
The real world is more complex. Advertisers, or more broadly, data lobbyists, aren’t picking between sharing two datapoints, but a near infinite set. They’re also choosing between messages with datapoints (“George won $8M”) and more qualitative ones (a video ad of a new friendship starting when a person gifts their neighbor a lottery ticket over the holidays).
A Clarification
I think it’s important to note that the data lobbyist isn’t necessarily out to get the data lobbied to act against their own best interests. They’re interested in getting the data lobbied to take an action that benefits the lobbyist. It’s possible there’s an action that benefits or is optimal for them both.
A financial advisor with a long track record of success can’t get new clients without putting in at least a little effort. They’ll need to put together information on their services, which includes data, to present to new clients to win their business. This is also data lobbying, however, in this case the decision to select this expert financial advisor benefits both the advisor and their prospective client.
Ok, so?
If you’ve used data to make correct decisions personally or professionally, none of the aforementioned should come as a surprise. You’re aware that individuals and organizations have unique incentives, and sometimes those incentives are in conflict. It makes sense that an entity trying to persuade another will share information, including data, in order get the consumer of that information to take an action that benefits the information sharer. In order to become resilient to data lobbying, decision makers need to independently determine what information they need to make a decision, acquire it4, process it, and then act on the results. Those who are skilled at this are quick to discard or discount data presented to them by somebody whose incentives may be in conflict with their own. For example, a salesperson wants to sell me their company’s product and provides me with information that encourages me to do that, but I’m interested in buying the product that best serves my needs, which may or may not be theirs.
This post is meant to be a simple introduction to the idea of data lobbying. I’ll explore this idea further in a subsequent post on a more complex type of data lobbying problem that data science organizations frequently encounter involving causal inference. I’ll follow that one with an examination of the incentives of data scientists and5 those they work with, and their implications on proper organizational design.
I started this newsletter in part to encourage more discussions and knowledge sharing around data strategy. I’d love to get your feedback, questions, and comments!
I caveat in that post that I’m viewing data in an applied sense.
To those I say, if you want something done right, do it yourself.
Or are victims of, if you’re making a value judgment.
Often these data are not readily available as data lobbyists don’t tend to share information that hurts their chances of getting what they want.
Or vs., spoiler alert.